Table Of Content

As one might imagine, there also are various designs and approaches that range between the end points of immediate experience and experiences aggregated over the entire interval. These intervals obviously are close together in time, and therefore are conceptually similar to one’s immediate state; nevertheless, they do require both increased levels of recall and some degree of mental aggregation. Similarly, studies with a longer time interval (e.g., 6-months) might nevertheless ask about one’s relatively recent experiences (e.g., affect over the past week), requiring less in terms of recall and mental aggregation, but only partially covering the events of the entire intervening interval. As a consequence, these two approaches and the many variations in between form a continuum of abstraction containing a number of differences that are worth considering.
Disadvantages of longitudinal studies

For example, performances might affect self-efficacy beliefs such that self-efficacy beliefs end up aligning with performance levels. This is why the multiple wave measurement practice is so important in passive observational panel studies. Because any single wave of a longitudinal design is itself cross-sectional data, a moratorium is not technically possible. This recommendation is tantamount to a moratorium on cross-sectional research papers, because almost all theories imply the lagged and/or longitudinal parameters in Figure 2.
Longitudinal vs cross-sectional studies
Researchers in this study have followed the same men group for over 80 years, observing psychosocial variables and biological processes for healthy aging and well-being in late life (see Harvard Second Generation Study). If you started out wondering what is a longitudinal study, we hope that we’ve addressed that question and more in this article. If you want to create a longitudinal survey, don’t forget to first plan out your survey.
Outcomes prediction in longitudinal data: Study designs evaluation, use case in ICU acquired sepsis - ScienceDirect.com
Outcomes prediction in longitudinal data: Study designs evaluation, use case in ICU acquired sepsis.
Posted: Wed, 10 Mar 2021 07:09:22 GMT [source]
Panel Study
The slope of this new variable represents the increment (up or down) to what the slope would have been had the individuals not been contacted by a recruiter. If it is statistically nonsignificant, then there is no change in slope pre- versus post-recruiter contact. If it is statistically significant, then the slope after contact differed from that before the contact. Finally, while much of the above is based upon a multilevel approach to operationalizing change, Muthén and Muthén (1998–2012) offer an SEM approach to time-varying covariates through their Mplus software package. In some cases, longitudinal researchers will wish to know the nature and dynamics of one’s immediate experiences. In these cases, the items included at each point in time will simply ask participants to report on states, events, or behaviors that are relatively immediate in nature.
Smart Surveys
For example, PACO is a (currently) free Google app that is in the beta-testing stage and allows great flexibility in the design and implementation of repeated surveys on both Android OS and iOS smartphones. Another example that is currently being developed for both Android and iOS platforms is Expimetrics (Tay, 2015), which promises flexible design and signaling functions that is of low cost for researchers collecting ESM data. Such applications offer the promise of highly accessible survey administration and signaling and have the added benefit of transmitting data quickly to servers accessible to the research team. Ideally, such advances in accessibility of survey administration will allow increased response rates throughout the duration of the longitudinal study.
I believe it was these kinds of designs that Ployhart and Vandenberg (2010) had in mind when they created their definition of longitudinal research. In particular, these designs can be used to assess rates of change and can improve causal inferences if done well. In particular, to improve causal inferences with panel designs, researchers nearly always need at least three repeated measures of the hypothesized causes and effects.
Longitudinal studies allow social scientists to distinguish short from long-term phenomena, such as poverty. If the poverty rate is 10% at a point in time, this may mean that 10% of the population are always poor or that the whole population experiences poverty for 10% of the time. Latent growth curve models allow researchers to model intraindividual change over time. For example, one could estimate parameters related to individuals’ baseline levels on some measure, linear or nonlinear trajectory of change over time, and variability around those growth parameters. Important methodological considerations include testing measurement invariance of constructs across time, appropriately handling missing data, and using accelerated longitudinal designs that sample different age cohorts over overlapping time periods. My hope or wish for the next big thing is the use of longitudinal methods to integrate the micro and macro domains of our literature on work-related phenomena.
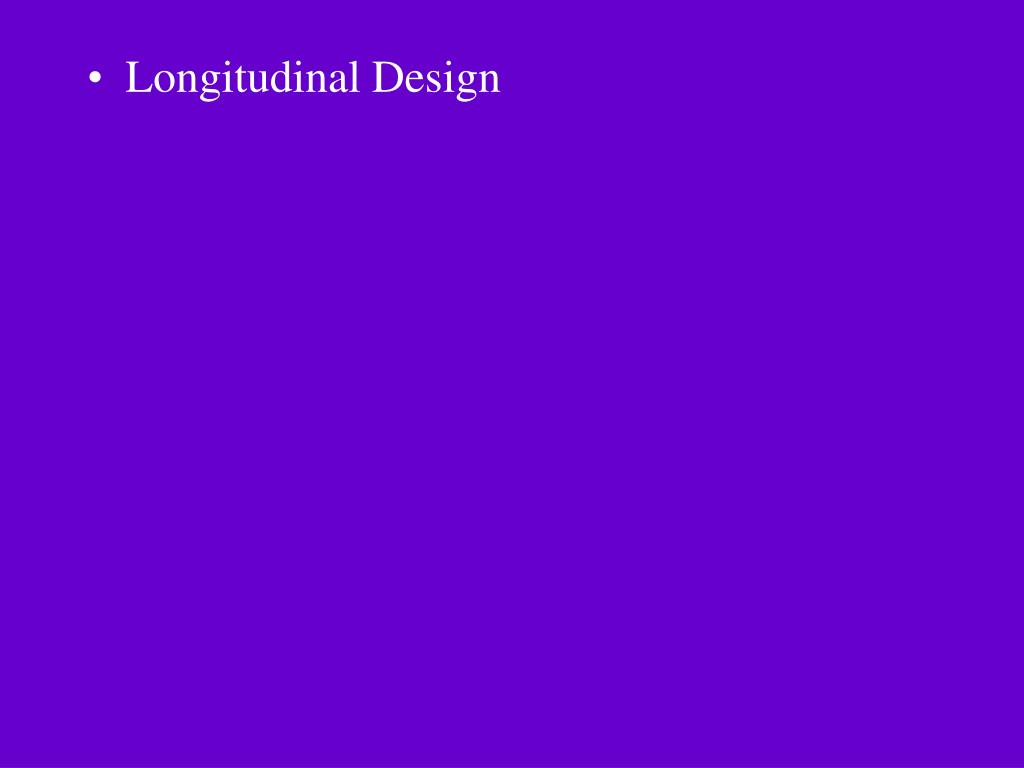
Longitudinal Research Designs
It is essential that the methods of data collection and recording are identical across the various study sites, as well as being standardised and consistent over time. Data must be classified according to the interval of measure, with all information pertaining to particular individuals also being linked by means of unique coding systems. Recording is facilitated, and accuracy increased, by adopting recognised classification systems for individual inputs (2). A longitudinal study is a type of observational and correlational study that involves monitoring a population over an extended period of time. Observing the same set of people can make sure that what you’re observing is a change over time. Visualizing the change over time will give you a clear idea of the trends and patterns, resulting in informed and effective decision-making.
Collecting your own data
Prospective longitudinal studies eliminate the risk of recall bias, or the inability to correctly recall past events. This means you can better establish the real sequence of events, allowing you insight into cause-and-effect relationships. Retrospective studies are generally less expensive and take less time than prospective studies, but they are more prone to measurement error. You then decide to design a longitudinal study to further examine this relationship in men. Without the cross-sectional study first, you would not have known to focus on men in particular.
In a prospective design, some criterion (i.e., presumed effect) is measured at Times 1 and 2, so that one can examine change in the criterion as a function of events (i.e., presumed causes) happening (or not) between the waves of data collection. For example, a researcher can use this design to assess the psychological and behavioral effects of retirement that occur before and after retirement. That is, psychological and behavioral variables are measured before and after retirement. Though not as internally valid as an experiment (which is not possible because we cannot randomly assign participants into retirement and non-retirement conditions), this prospective design is a substantial improvement over the typical design where the criteria are only measured at one time. This is because it allows one to more directly examine change in a criterion as a function of differences between events or person variables.
As noted, dynamic or random walk variables can create problems for poorly designed longitudinal research because one may not realize that the level of the criterion (Y), say measured at Time 3, was largely near its level at Time 2, when the presumed cause (X) was measured. Moreover, at Time 1 the criterion (Y) might have been busy moving the level of the “causal” variable (X) to the place it is observed at Time 2. That is, the criterion variable (Y) at Time 1 is actually causing the presumed causal variable (X) at Time 2.
Central amongst these are (I) the linked nature of the data for an individual, despite separation in time; (II) the co-existence of fixed and dynamic variables; (III) potential for differences in time intervals between data instances, and (IV) the likely presence of missing data (6). It is important to note that the mean structure approach not only applies to longitudinal models with three or more measurement points, but also applies to simple repeated measures designs (e.g., pre–post design). Traditional paired sample t tests and within-subject repeated measures ANOVAs do not take into account measurement equivalence, which simply uses the summed scores at two measurement points to conduct a hypothesis test. The mean structure approach provides a more powerful way to test the changes/differences in a latent variable by taking measurement errors into consideration (McArdle, 2009). The latter quote serves as a segue to address the second part of our question, “Given that longitudinal research purportedly addresses the limitations of cross-sectional research, can findings from cross-sectional studies be useful for the development of a theory of change? ” Obviously, the answer here is “it depends.” In particular, it depends on the design contexts around which the cross-sectional study was developed.